- unwind ai
- Posts
- No-code IDE to Build Multi-agent Systems with MCP
No-code IDE to Build Multi-agent Systems with MCP
PLUS: Opensource multimodal RAG running locally, Test your AI with 1000s of digital humans
Today’s top AI Highlights:
Open-source RAG that understands PDF images, runs locally
No-code AI IDE to build multi-agent systems with MCP tools
AI agents in a long-term vending machine business
Open-source 1.6B text-to-speech model outperforming Eleven Labs and Sesame
Test your AI with 1000s of digital humans
& so much more!
Read time: 3 mins
AI Tutorial
Financial management is a deeply personal and context-sensitive domain where one-size-fits-all AI solutions fall short. Building truly helpful AI financial advisors requires understanding the interplay between budgeting, saving, and debt management as interconnected rather than isolated concerns.
A multi-agent system provides the perfect architecture for this approach, allowing us to craft specialized agents that collaborate rather than operate in silos, mirroring how human financial advisors actually work.
In this tutorial, we'll build a Multi-Agent Personal Financial Coach application using Google’s newly released Agent Development Kit (ADK) and the Gemini model. Our application will feature specialized agents for budget analysis, savings strategies, and debt reduction, working together to provide comprehensive financial advice. The system will offer actionable recommendations with interactive visualizations.
We share hands-on tutorials like this every week, designed to help you stay ahead in the world of AI. If you're serious about leveling up your AI skills and staying ahead of the curve, subscribe now and be the first to access our latest tutorials.
Latest Developments
Rowboat is a Cursor-like AI-assisted no-code IDE for building multi-agent assistants powered by OpenAI's Agents SDK. The platform features a visual interface where you can create agents using plain language instructions. Rowboat's AI copilot handles the complex work of generating agents based on your requirements, while still giving you the option to configure everything manually.
With built-in MCP tool integration and RAG capabilities, you can quickly develop and deploy multi-agent systems that access external data sources and tools.
Key Highlights:
AI builds AI Agents - Describe what you need in everyday language, and Rowboat's copilot handles the technical work of creating appropriate agents, setting up instructions, and configuring connections between them. The copilot remains context-aware of all components and can refine agents based on test conversations.
MCP Tools - Import tools directly from any MCP server through a simple settings interface. Connect these tools to specific agents to extend their capabilities, with options to mock tool responses during testing or integrate production tools via webhooks.
Knowledge Integration with RAG - Equip your agents with domain knowledge using RAG. It can readily integrate with your existing Elasticsearch or embedding-based retrieval systems for improved grounding.
Production-Ready - Move from prototype to production with the built-in HTTP API and Python SDK. The platform supports both stateful and stateless conversation management, making it easy to integrate your assistants into websites, applications, or backend systems.
Morphik is an open-source multimodal RAG system that solves the persistent problem of extracting information from images and diagrams embedded in PDFs. Even state-of-the-art LLMs like GPT-4o and o3 fail to accurately interpret visual data from technical documents, missing out on crucial information in charts, tables, and diagrams.
Morphik uses Colpali-style embeddings that treat each document page as an image and generate multi-vector representations. These embeddings capture layout, typography, and visual context, allowing the system to retrieve entire tables or schematics instead of just text fragments. With this capability, even an 8B Llama 3.1 vision model running locally can answer complex visual queries that stumped much larger models.
Key Highlights:
True Multimodal Understanding - Besides just text, Morphik actually comprehends visual content in documents. It embeds entire pages as images to preserve context, allowing it to retrieve relevant diagrams, charts, and tables without complex preprocessing pipelines.
Knowledge Graph Integration - Morphik builds knowledge graphs by tagging entities in both text and images, normalizing synonyms, inferring relations, and connecting everything into a searchable structure. This enables cross-document queries by traversing connections between related concepts.
Performance Optimization - The system implements persistent KV caching that stores intermediate key-value states from transformer attention layers, allowing it to reuse prior computations rather than recalculating attention from scratch. This improves handling of much longer context windows without performance degradation.
Dev-Friendly - Morphik provides simple APIs for document ingestion and querying, with features like natural language rules for data processing, user and folder scoping for organization, and flexible model registry for using different AI models based on task complexity. Everything is completely open-source.
Quick Bites
AI agents show impressive performance in complex isolated short-term tasks but slip into bad plans and memory gaps when tasks are simple but long-running. Here's a benchmark to assess just this capability of agents.
Sweden-based Andon Labs has open-sourced Vending-Bench that puts an AI agent into a simulated vending-machine business—buying stock, setting prices, and covering daily fees—over runs that burn roughly 20-25 million tokens. The agents need to handle ordering, inventory management, and pricing over long horizons to successfully make money. Early evals show that Claude 3.5 and 3.7 Sonnet and o3-mini made the strongest profit while several rivals went broke. The code is open-source, so clone the repo, plug in your model key, and see how it fares.
In a cluttered market of text-to-speech models, this small 1.6 billion parameter open-source model outperforms competitors like Sesame and ElevenLabs. Dia 1.6B is a text-to-speech model by two Korea-based undergrads who started Nari Labs with little to no experience in speech AI. This model directly generates very human-like dialogue from transcripts with customizable emotions, voice cloning capabilities, and even non-verbal communications like laughter and coughing. The model is now available on Hugging Face; do check out the demos!
Just like OpenAI’s Advanced Voice Mode, xAI has released vision capabilities, multilingual audio, and real-time web search capabilities in Grok’s voice mode. It is now available to all Grok users on iOS app and SuperGrok users on Android.
Tools of the Trade
Microagents: Create small, task-specific AI agents that work together inside a group chat. You connect your apps (like Gmail, Notion, Slack), set up agent teams, and assign tasks—these agents then collaborate in real-time to complete the work.
Ghostrun: A unified API that lets you switch between multiple AI model providers using one interface, with threading to keep context across calls. It also offers built-in RAG pipelines, handles all API key and payment management, and exposes provider pricing without adding any extra cost.
Autoblocks AI: Test your agentic application with thousands of simulated real-world user interactions—including voice calls, edge cases, and noisy conditions—to evaluate and improve AI agents before production. It auto-generates test cases, flags weak spots in conversational logic, and integrates with your existing stack for continuous monitoring.
Awesome LLM Apps: Build awesome LLM apps with RAG, AI agents, and more to interact with data sources like GitHub, Gmail, PDFs, and YouTube videos, and automate complex work.
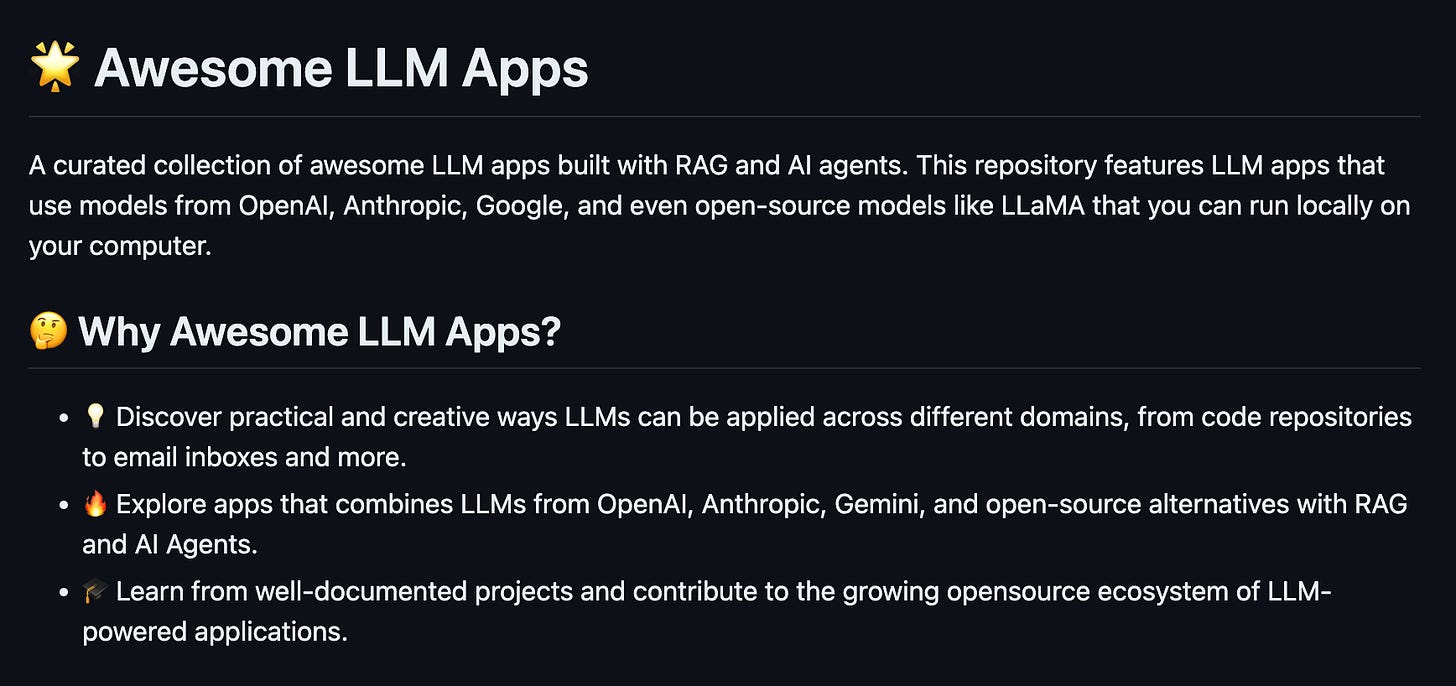
Hot Takes
"everyone just agree to not use AI in situations where it would be personally beneficial to do so" is not a particularly stable equilibrium ~
will brownCoding models basically don't work if you're building anything net new. Vibe coding only works when you split down a large project into components likely already present in the training dataset of coding models. ~
Sherjil Ozair
That’s all for today! See you tomorrow with more such AI-filled content.
Don’t forget to share this newsletter on your social channels and tag Unwind AI to support us!
PS: We curate this AI newsletter every day for FREE, your support is what keeps us going. If you find value in what you read, share it with at least one, two (or 20) of your friends 😉
Reply